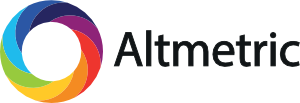
Why do some of us choose to remain ignorant of information that – though perhaps unpleasant – could help us make better informed decisions in the future? In episode 76, Emily Ho from Northwestern University’s Department of Medical Social Sciences discusses her research into why we keep our heads in the sand about important information for a variety of psychological and economic reasons. Her article “Measuring information preferences,” was published on March 13, 2020 with David Hagmann and George Loewenstein in the journal Management Science.
Websites and other resources
-
- Emily’s website and Twitter feed
- Take Emily’s Information Preferences Scale yourself!
Bonus Clips
🔊 Access bonus content here.
Support us for as little as $1 per month at Patreon. Cancel anytime.
We’re not a registered tax-exempt organization, so unfortunately gifts aren’t tax deductible.
Hosts / Producers
Ryan Watkins & Doug Leigh
How to Cite
Music
What’s The Angle? by Shane Ivers